Recently, the research work titled "Message-Driven Generative Music Steganography Using MIDI-GAN" by Associate Professor Zhaopin Su from the School of Computer Science and Information Engineering at Hefei University of Technology has been officially published in IEEE Transactions on Dependable and Secure Computing (TDSC), a top-tier international journal in the field of network and information security. The corresponding author of the paper is Professor Guofu Zhang.
TDSC is one of the most influential journals in the domain of network and information security, focusing on the reliability, security, and availability of computer systems and networks. It is recognized as a CCF Class A journal. The journal regularly publishes high-quality research papers on topics such as computer and network security, trusted computing, reliability analysis, fault-tolerant systems, and distributed systems. It is widely regarded as a leading journal in computer science, with publications often representing the latest advancements and highest standards in the field.
Paper Title: Message-Driven Generative Music Steganography Using MIDI-GAN
Authors: Zhaopin Su; Guofu Zhang; Zhiyuan Shi; Donghui Hu; Weiming Zhang
Link to the Paper: https://ieeexplore.ieee.org/document/10457038
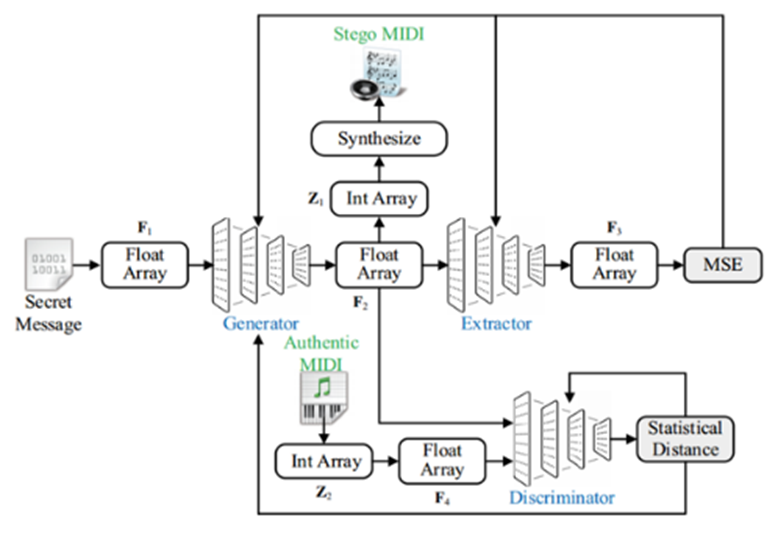
Figure 1: The Basic Architecture of MIDI-GAN
The generator is responsible for directly converting secret information into steganographic MIDI files. The discriminator aims to make the steganographic MIDI files as close as possible to real-life authentic MIDI files. The extractor is tasked with retrieving the secret information from the steganographic MIDI files or chord sequence numbers.
A message-mapping mechanism combined with error correction codes (ECC) is proposed to convert binary secret messages into floating-point arrays for synthesizing MIDI files. Notably, the proposed mechanism ensures that the same bit or bits are mapped to entirely different chords each time, which enhances resistance to steganalysis. A GAN-based message-driven generative MIDI steganography scheme, called MIDI-GAN, is developed to evade detection by deep learning-based steganalysis. In MIDI-GAN, the generator converts secret information into artificially generated steganographic MIDI files that are compact, melodious, and undetectable by steganalysis tools. Uniquely, these steganographic MIDI files can also be transmitted in the form of chord sequences, distinguishing them from existing generative image/audio steganography techniques and providing no grounds for suspicion. Furthermore, the discriminator ensures that the steganographic MIDI files closely resemble authentic MIDI files. The extractor is designed to recover the secret messages from the steganographic MIDI files or chord sequences. In MIDI-GAN, adversarial learning is implemented between the generator and the discriminator, as well as between the generator and the extractor. This enhanced adversarial training allows the generator, discriminator, and extractor to compete and evolve together, leading to improved concealment, undetectability, and robustness.